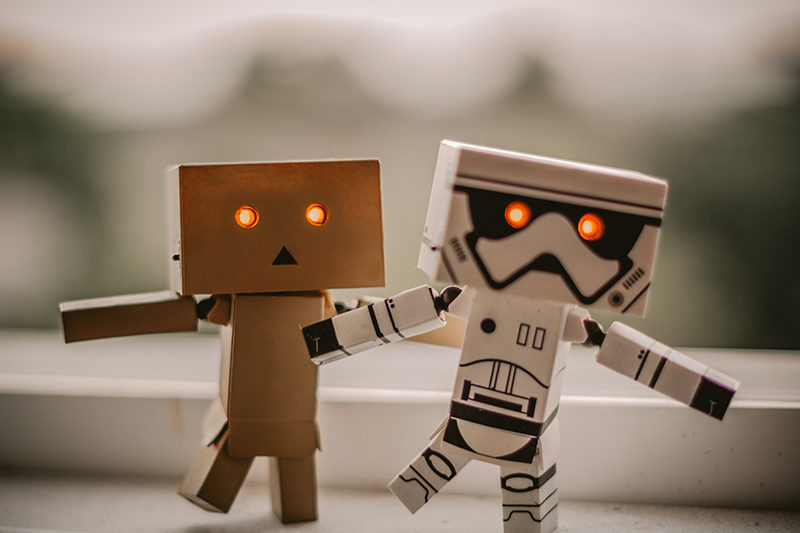
Arnold Schwarzenegger and the fictional Terminator movie franchise popularized the idea of machines surpassing human intelligence, and we all laughed (or cried, depends on who's side you were on in the movie).
However, in today's world, this fictional story of machines being able to "think"
and "learn" is now a reality. Artificial Intelligence (AI) and Machine Learning
(ML) are behind some of today's most popular technologies. Take for example
Google's Assistant who recently called and made a reservation at a hair salon (View it here) in such a manner that myself and
the person on the other end of the phone thought it was a human being. Scary,
huh?
The opposite side of the coin is the use of AI and ML in
ride-sharing apps such as Uber and Lyft. Both technologies are used to minimize
your wait time by analyzing which drivers are closest to you. Additionally, they
are used to determine the cost of your ride, based on the demand for trips at
any given time. Without these two intertwined technologies, we would be stuck
taking public transportation.
Auto-pilot on planes, spam filters in
email software, mobile check deposits to your banks, these all utilize the twin
technologies of AI and ML.
What is
Artificial Intelligence and Machine
Learning?
Artificial Intelligence and Machine
Learning is a way of making a computer think intelligently. A computer program
without AI or ML can answer specific questions, with pre-programmed responses.
However, equipment with AI and ML can not only return pre-programmed answers but
can learn new information and return it to the end user.
In 2008 Apple
used flash memory to increase the number of songs consumers could
download to their iPods. The use of flash memory was a significant deviation
from previous thinking that only hard disk drives could operate fast enough to
be useful. Within one year EMC, Sun Microsystems, Intel, and Hitachi began
championing this upstart technology for business users. With the increase of
speed in processing also came a drastic reduction in space necessary to house
the memory.
The use of flash memory allowed software programs to run
faster and with the inclusion of new hardware to run these quicker processes.
Artificial Intelligence was soon on the horizon. Because of the speed inherent
in flash memory, a computer could now take in exponentially more data than
previously dreamed of and return an answer in a fraction of the time than before
2008.
The access to billions of megabytes of data, and the speed
necessary to process that data quickly is, at heart, what is Artificial
Intelligence. Simply put, AI is the ability of a computer to take in a question
or a set of variables and query its database at human thought-like speeds and
return the most statistically probable answer.
Machine Learning is the
ability of that computer to use the questions and answers from Artificial
Intelligence and update its databases based on this new input to offer new
solutions or share more background information.
A modern-day example of
this process is the Google Assistant. This device has access to all of Google's
search databases and can interpret your request, send a query to these
databases, find the correct answer, and return it to the device, translate it
into language, and speak the response to you with a second or two. What is more
impressive is that this household device can add to its databases without
specific programming based on new inquiries to "learn" new things such as the
score of last night's Yankees' game.
So how do Artificial Intelligence and Machine
Learning affect the world of radiology and healthcare?
Whether you know it or not there are already some companies in the
healthcare industry that offer automated analysis and reporting for pulmonary,
cardiology, and radiology patients. There are also companies that provide
automated image searches for radiologists to assist in comparing similar cases
for better diagnosis. Recently, industry voice Aunt Minnie announced its
“Minnies 2018 Semifinal Awards Candidates.*” Artificial
Intelligence is represented in the categories “Most Significant News Event”,
“Biggest Threat to Radiology”, and in numerous offerings in “Best New Radiology
Software.”
These modern technologies may one day assist a radiologist in
finding a tiny nodule in a patient’s lung, or a minuscule fracture in a child's
femur. Together, these advancements in medical imaging diagnostics will further
increase the level of healthcare for patients.
However, with the rise of
AI and ML in today's society many fear the repercussions of "Monday Morning
Quarterbacking" with regards to diagnoses.
Let's say, Patient John goes
to XYZ Hospital because his wrist was hurt playing softball. The physicians at
XYZ hospital order X-rays, the X-rays are read by a physician and run through
XYZ Hospitals' AI database, and the diagnosis is that there are no breaks to
John's wrist. After six months, Patient John is still experiencing some pain in
the injured wrist and goes to ABC Hospital. Once again, X-rays are ordered, and
the physician does not see any fracture but runs the X-ray through the
hospital's AI database. ABC Hospital's AI database compares Patient John's X-ray
to all of its similar X-rays and returns a diagnosis of a fracture based on a
similar case from less than a month ago.
Would this scenario open the
physician and XYZ Hospital to malpractice? In today's litigious society, could
an attorney make a case that XYZ Hospital should have used a different set of AI
databases?
There are currently no American Medical Association standards
for diagnoses for Artificial Intelligence. As of June 14, 2018, the AMA has
adopted a policy that will:**
- Leverage its ongoing engagement in digital health and other priority areas for improving patient outcomes and physicians’ professional satisfaction to help set priorities for health care AI.
- Identify opportunities to integrate the perspective of practicing physicians into the development, design, validation, and implementation of health care AI.
- Promote development of thoughtfully designed, high-quality, clinically
validated health care AI that:
- is designed and evaluated in keeping with best practices in user-centered design, particularly for physicians and other members of the health care team
- is transparent
- conforms to leading standards for reproducibility
- identifies and takes steps to address bias and avoids introducing or exacerbating health care disparities including when testing or deploying new AI tools on vulnerable populations
- patients’ and other individuals’ privacy interests and preserves the security and integrity of personal information
- Encourage education for patients, physicians, medical students, other health care professionals, and health administrators to promote greater understanding of the promise and limitations of health care AI.
- Explore the legal implications of health care AI, such as issues of liability or intellectual property, and advocate for appropriate professional and governmental oversight for safe, effective, and equitable use of and access to health care AI.
Meanwhile, the FDA has recently proposed changing AI products from the more
restrictive class III category to category II. This proposal would place all AI
software into the same category as CTs, Radiography Rooms, and MRI scanners.
This move would allow AI and ML software quicker entry into the market by
reducing the amount of research and testing necessary to bring a product to
market.
As Artificial Intelligence and Machine Learning continue to
evolve, I am sure that there will be innovations that will improve healthcare.
However, until governing agencies have the opportunity to perform clinical
trials, conduct peer review, and evaluate findings, let us all continue to learn
and use our best judgments to assess the level of AI and ML that we allow into
our professional lives.
"Hasta la vista, baby."
- * “Meet the Minnies 2018 Semifinal Candidates.” AuntMinnie.com, AuntMinnie.com, 28 Aug. 2018, www.auntminnie.com/index.aspxsec=nws&sub=rad&pag=dis&ItemID=121692.
- ** “AMA Passes First Policy Recommendations on Augmented Intelligence.” HIPAA Compliance | American Medical Association, 14 June 2018, www.ama-assn.org/ama-passes-first-policy-recommendations-augmented-intelligence.
Comments
Rachel D.
Orlando, FL
Great Article
Thank you for the article. You've made complex concepts easy to follow. The Google thing is very scary. I am excited to see where AI and ML bring in the future.
Friday, September 28, 2018Leave a Comment